AI is being successfully used by a select group of companies to enhance everything from customer experiences to operational efficiency.
These AI champions are seeing tangible results, gaining competitive advantages, and transforming their industries.
However, most companies are still struggling with pilot projects or limited AI deployments and are not able to scale their initiatives effectively or demonstrate measurable results.
What can companies do to move beyond AI experimentation and integrate AI into their core operations while also measuring the impact of their investments?
Enter AI governance – the linchpin that can make or break your AI strategy.
In this post, we’ll explore why governance is crucial for measuring and maximizing the impact of AI initiatives and how you can establish a model that works for your unique organizational context.
Why Governance Matters in AI Initiatives
Think of AI governance as the conductor of an orchestra.
It’s the framework of policies, processes, and structures that ensures all the instruments (or in this case, AI technologies) work in harmony. But it’s more than just keeping things in check – it’s about fostering an environment where AI can flourish and deliver real, tangible value to your business.
Why does governance matter for AI success?
Simply put, it provides the necessary guardrails and accountability to ensure that AI initiatives align with business objectives, comply with ethical and regulatory standards, and deliver measurable results. Without proper governance, AI projects risk becoming expensive experiments with little real-world impact.
The link between governance and return on AI investment is clear.
Our research at the Return on AI Institute has shown that organizations with well-established AI governance models are better positioned to demonstrate the value of their AI initiatives, secure ongoing investment, and scale successful projects across the enterprise.
3 Approaches to Measuring AI Impact
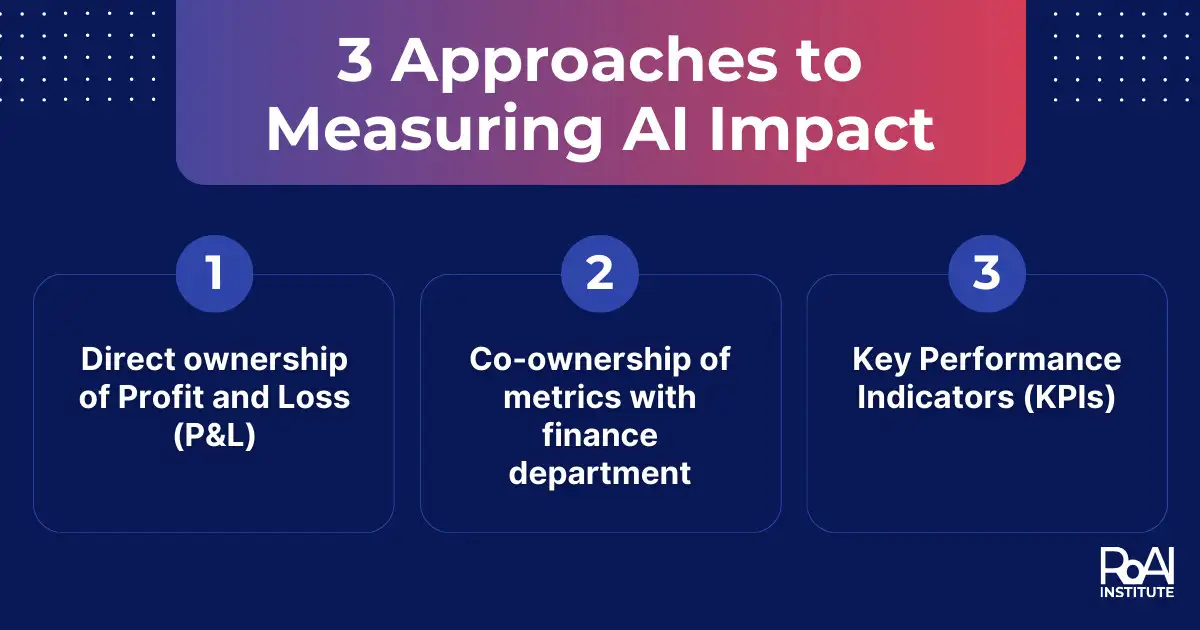
Through our extensive study of AI initiatives across various industries, we’ve identified three primary models for AI governance:
1. Direct ownership of Profit and Loss (P&L)
In this approach, the AI team is directly accountable for revenue and profit targets. It’s a high-stakes, high-reward approach that gives AI leaders full control over not just the AI models but also the business processes they impact.
Benefits:
- Clear accountability and direct attribution of results
- Ability to quickly iterate and optimize AI solutions
- Full control over end-to-end processes
Challenges:
- May limit the potential for enterprise-wide transformation
- Requires significant organizational buy-in
- Can create siloes if not managed carefully
2. Co-ownership of metrics with finance department
This approach involves a sort of “shadow P&L,” typically structured around initiative costs and value measurement. It’s a more collaborative model that often fits well in matrix organizations.
Benefits:
- Encourages cross-functional collaboration
- Balances AI expertise with financial rigor
- Can facilitate broader adoption across the organization
Challenges:
- Potential for diffused accountability
- May slow decision-making processes
- Requires strong alignment between AI and finance teams
3. Key Performance Indicators (KPIs)
In this model, the AI team is accountable for specific functional outcomes – think metrics like customer call response times or inventory turnover rates.
Benefits:
- Clear, measurable objectives
- Easier to align with existing business metrics
- Can be more intuitive for non-technical stakeholders
Challenges:
- May not capture the full value of AI initiatives
- Risk of focusing on short-term wins over long-term transformation
- Can lead to a narrow focus on specific use cases
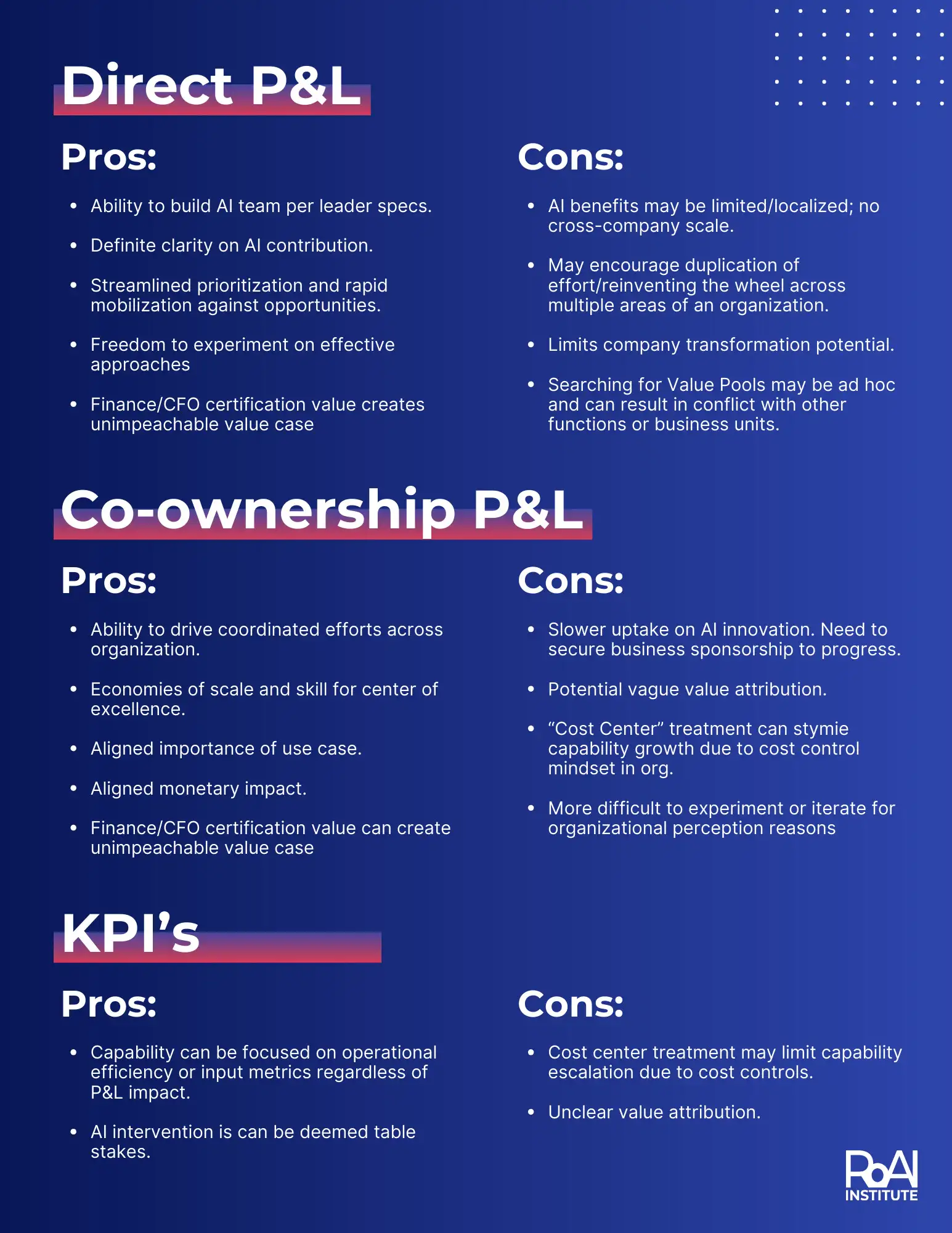
The Impact of Organizational Culture on Governance Model Selection
As management guru Peter Drucker famously said, “Culture eats strategy for breakfast.” When it comes to AI, we might say, “Culture eats AI for breakfast.”
Our research has shown that the way companies measure the return or impact of AI is largely determined by how they manage everything else.
Understanding existing processes and workflows is crucial.
For instance, in our case study of a Fortune 100 retailer, the AI leader spent time working night shifts in stores to truly grasp the nuts and bolts of the business. This deep understanding allowed him to identify high-value AI opportunities and implement them effectively.
Adapting AI initiatives to fit organizational culture is key to success. The governance model you choose should align with your company’s existing decision-making processes, performance management practices, and overall business philosophy.
4 Factors Influencing the Choice of Governance Model
Several factors come into play when selecting the right governance model:
- Organizational culture
- Does your company favor centralized or decentralized decision-making?
- How is performance typically measured and rewarded?
- Existing performance management practices
- Are you already using balanced scorecards, OKRs, or other frameworks?
- Your AI governance should complement these existing systems.
- Nature and scope of AI projects
- Are you focusing on narrow, department-specific use cases, or aiming for enterprise-wide transformation?
- Stage of AI journey
- Early-stage experimentation might require different governance than mature, scaled AI operations.
Implementing Your AI Governance Model
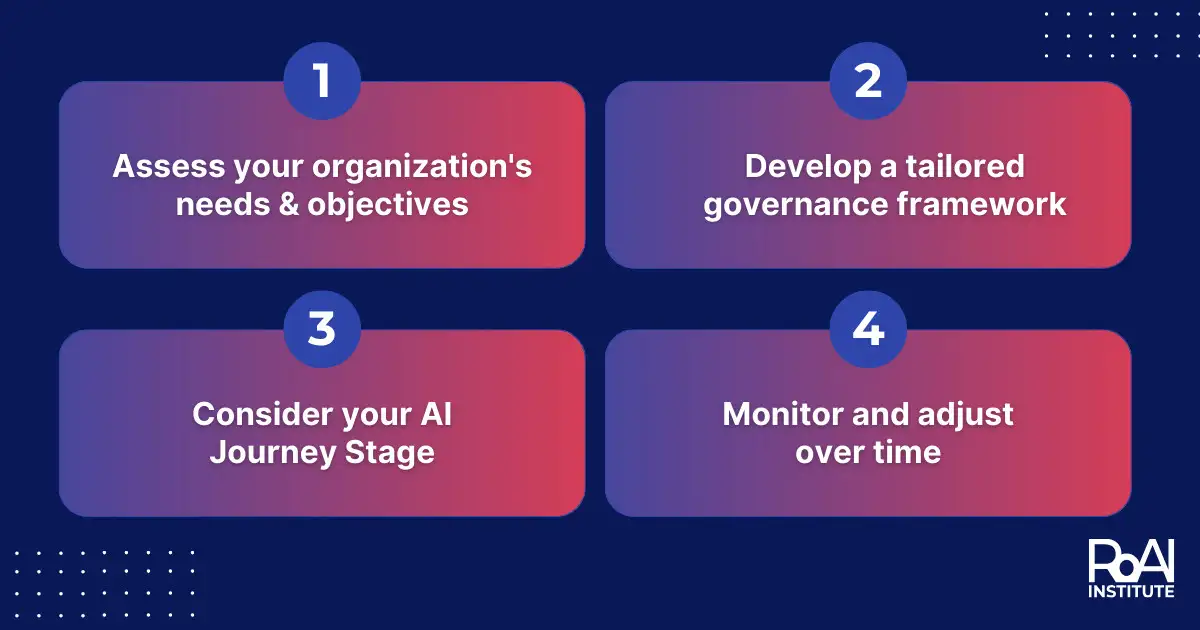
Now that we’ve explored the various aspects of AI governance, you might be wondering: “How do I put this into practice?”.
Here are four key steps to guide you through the process of establishing and maintaining a governance framework that drives measurable AI impact:
- Assess your organization’s needs & objectives
- Conduct a thorough analysis of your current AI capabilities, business goals, and organizational culture.
- Develop a tailored governance framework
- Based on your assessment, choose the model that best fits your organization.
- Consider your AI journey stage
- Early-stage experimentation might require different governance than mature, scaled AI operations.
- Monitor and adjust over time
- Regularly review the effectiveness of your governance model and be prepared to evolve it as your AI initiatives mature.
Conclusion
The divide between AI leaders and laggards is growing wider by the day.
The differentiating factor?
It’s not just about having the most advanced algorithms or the largest datasets. The true key to AI success lies in effective governance. Effective AI governance isn’t about constraining innovation – it’s about creating an environment where AI can thrive and deliver tangible business value.
It’s the framework that allows organizations to move beyond pilot projects and limited deployments to achieve scalable, impactful AI initiatives across the enterprise.