Our research shows that less than 10% of companies have analytical proficiency at
the start of their AI journey.
Your company’s Analytical Quotient (AQ) will determine your ability to leverage AI. This article will help you assess your company’s analytical quotient and the steps to improve.
Understanding and Assessing Your Company’s Analytical Quotient (AQ)
Analytical Quotient (AQ) refers to an organization’s level of analytical proficiency, systems workflow, and quantitative experimentation mindset.
Based on research conducted by the Return on AI Institute, organizations typically fall into one of three AQ levels. Understanding these levels and their characteristics can help you assess your company’s performance and identify areas for improvement.
Strike Ready
Characteristics:
- The organization is inherently data-driven.
- Analytical methods and quantitative decision-making are routinely practiced.
- Talent across the organization possesses analytics and data science experience.
Assessment indicators:
- Teams can “do the work” without needing to “sell the why” of data-driven approaches.
- There’s a fast track to proof of concept or value capture for AI and analytics projects.
- Decision-makers consistently rely on data and analytics to inform their choices.
Mobilizing
Characteristics:
- The organization is willing to change and embrace data-driven approaches.
- There’s high awareness and appreciation for data-based, quantitative decision-making.
- Intentional upskilling of the workforce is underway.
Assessment indicators:
- The organization needs AI leaders to point the way and guide the transformation.
- Early AI wins galvanize the organization and build momentum.
- There’s a growing appetite for data-driven insights, but implementation may be inconsistent.
Bootcamp
Characteristics:
- The organization may be traditional or legacy-behavior-driven.
- Decision-making is highly dependent on hierarchical wisdom.
- Data and quantitative methods are under-leveraged.
Assessment indicators:
- The environment is challenging for AI leaders, who often feel isolated.
- There’s a significant need to convince the organization to change its approach to decision-making.
- AI and analytics initiatives face skepticism and resistance.
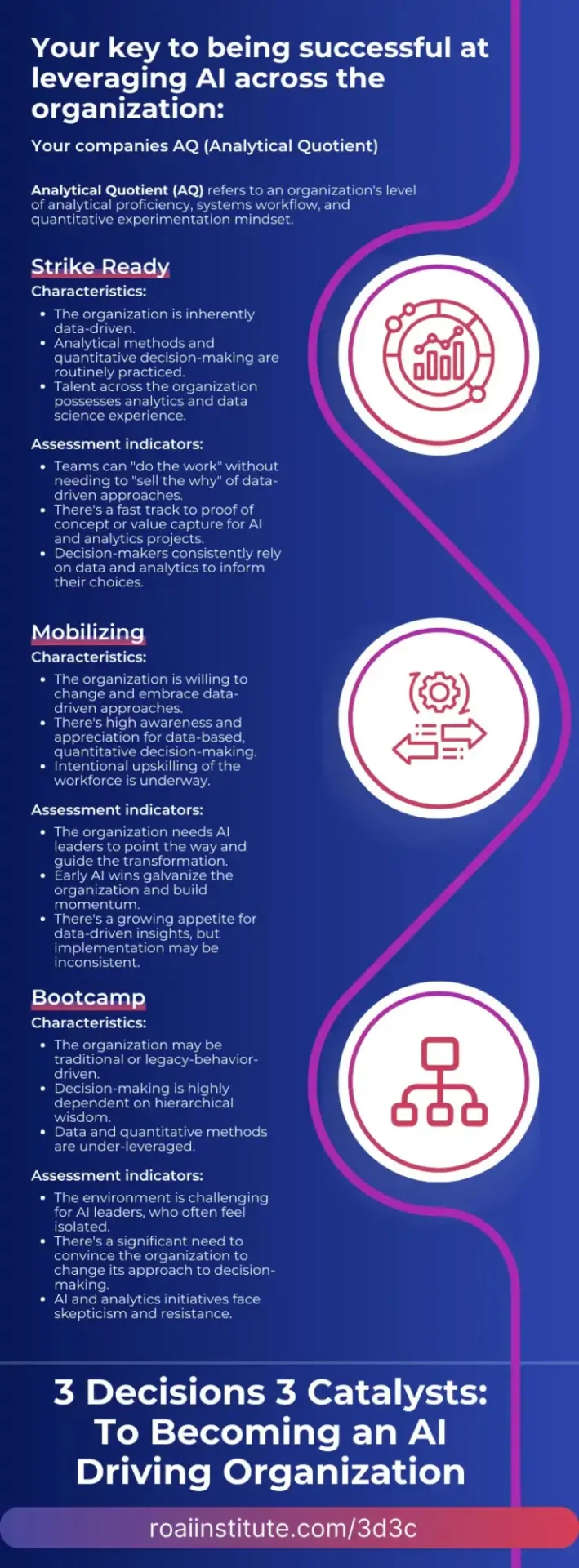
To assess your company’s current AQ level, consider the following questions:
- How are major decisions typically made in your organization? Are they based on data and analytics or rely more on intuition and experience?
- What is the general attitude towards data and analytics among leadership and employees? Is there enthusiasm, skepticism, or indifference?
- How easily can teams access and use data for their decision-making processes?
- Is there a formal strategy for developing analytical capabilities across the organization?
- How quickly can your organization move from an analytics-based idea to a proof of concept?
- Do you have the necessary talent and skills in-house to perform advanced analytics and AI tasks?
- Is there a culture of experimentation and learning from data-driven insights?
By evaluating your organization against these criteria and questions, you can better understand your current AQ level. This assessment is a crucial first step in developing a strategy to improve your company’s analytical capabilities and build a more data-driven culture.
Many organizations start at the Bootcamp level and progress through Mobilizing to eventually become strike-ready. The key is to recognize your current state and commit to continuously improving your analytical capabilities.
4 Strategies for Improving Your Company’s AQ
Regardless of your current AQ level, there are several strategies you can employ to improve:
- Secure leadership commitment to data-driven decision-making: Organize a leadership workshop where executives are presented with case studies of successful data-driven decisions in your industry. Follow this with a commitment from each leader to integrate data analysis into at least one major decision in the coming quarter. This could involve using data to inform product development, market expansion, or resource allocation decisions.
- Implement formal training programs to upskill existing teams: Develop a company-wide “Data Literacy” program. This could include courses ranging from basic data interpretation to advanced analytics techniques.
For instance, marketing teams could learn about A/B testing and customer segmentation analytics, while operations teams might focus on predictive maintenance and supply chain optimization techniques. 3. Hire experienced external talent to fill critical capability gaps: Recruit a Chief Data Officer or Chief Analytics Officer to lead your organization’s data strategy. Additionally, bring data scientists and machine learning engineers to form a core analytics team.
These experts can drive advanced analytics projects and mentor existing staff, accelerating your organization’s overall analytical capabilities. 4. Foster a culture of experimentation and learning: Implement a “Data Challenge” program where teams are encouraged to use data to solve business problems. Provide resources, mentorship, and recognition for innovative solutions. This could lead to discoveries like new customer insights, process efficiencies, or product innovations.
Additionally, create a “safe-to-fail” environment where teams are encouraged to test hypotheses using data, even if not all experiments lead to immediate successes.
Implementing these strategies can create a comprehensive approach to improving your organization’s AQ. This is an ongoing process that requires consistent effort and adaptation as your company’s analytical capabilities evolve.
Conclusion
Developing your company’s Analytical Quotient is crucial for a data-driven culture and successful AI initiatives. Assess your current AQ level, implement improvement strategies, and foster a more data-driven culture across all levels of the organization. The long-term benefits include improved decision-making, increased efficiency, and enhanced competitiveness.